This story was written by Vandana Janeja, professor and interim chair of information systems, and first appeared in The Conversation
Predicting the coronavirus outbreak: How AI connects the dots to warn about disease threats
Canadian artificial intelligence firm BlueDot has been in the news in recent weeks for warning about the new coronavirus days ahead of the official alerts from the Centers for Disease Control and Prevention and the World Health Organization. The company was able to do this by tapping different sources of information beyond official statistics about the number of cases reported.
BlueDot’s AI algorithm, a type of computer program that improves as it processes more data, brings together news stories in dozens of languages, reports from plant and animal disease tracking networks and airline ticketing data. The result is an algorithm that’s better at simulating disease spread than algorithms that rely on public health data – better enough to be able to predict outbreaks. The company uses the technology to predict and track infectious diseases for its government and private sector customers.
Traditional epidemiology tracks where and when people contract a disease to identify the source of the outbreak and which populations are most at risk. AI systems like BlueDot’s model how diseases spread in populations, which makes it possible to predict where outbreaks will occur and forecast how far and fast diseases will spread. So while the CDC and laboratories around the world race to find cures for the novel coronavirus, researchers are using AI to try to predict where the disease will go next and how much of an impact it might have. Both play a key role in facing the disease.
However, AI is not a silver bullet. The accuracy of AI systems is highly dependent on the amount and quality of the data they learn from. And how AI systems are designed and trained can raise ethical issues, which can be particularly troublesome when the technologies affect large swathes of a population about something as vital as public health.
It’s all about the data
Traditional disease outbreak analysis looks at the location of an outbreak, the number of disease cases and the period of time – the where, what and when – to forecast the likelihood of the disease spreading in a short amount of time.
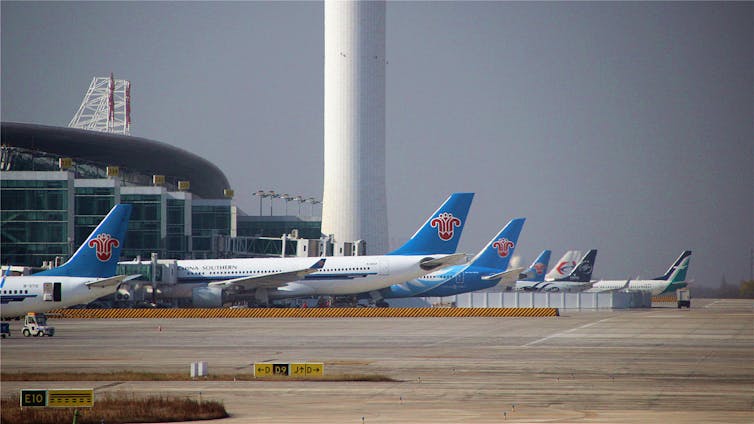
More recent efforts using AI and data science have expanded the what
to include many different data sources, which makes it possible to make
predictions about outbreaks. With the advent of Facebook, Twitter and
other social and micro media sites, more and more data can be associated
with a location and mined for knowledge about an event like an
outbreak. The data can include medical worker forum discussions about
unusual respiratory cases and social media posts about being out sick.
Much of this data is highly unstructured, meaning that computers can’t easily understand it. The unstructured data can be in the form of news stories, flight maps, messages on social media, check ins from individuals, video and images. On the other hand, structured data, such as numbers of reported cases by location, is more tabulated and generally doesn’t need as much preprocessing for computers to be able to interpret it.
Newer techniques such as deep learning can help make sense of unstructured data. These algorithms run on artificial neural networks, which consist of thousands of small interconnected processors, much like the neurons in the brain. The processors are arranged in layers, and data is evaluated at each layer and either discarded or passed onto the next layer. By cycling data through the layers in a feedback loop, a deep learning algorithm learns how to, for example, identify cats in YouTube videos.
Researchers teach deep learning algorithms to understand unstructured data by training them to recognize the components of particular types of items. For example, researchers can teach an algorithm to recognize a cup by training it with images of several types of handles and rims. That way it can recognize multiple types of cups, not just cups that have a particular set of characteristics.
Any AI model is only as good as the data used to train it. Too little data and the results these disease-tracking models deliver can be skewed. Similarly, data quality is critical. It can be particularly challenging to control the quality of unstructured data, including crowd-sourced data. This requires researchers to carefully filter the data before feeding it to their models. This is perhaps one reason some researchers, including those at BlueDot, choose not to use social media data.
One way to assess data quality is by verifying the results of the AI models. Researchers need to check the output of their models against what unfolds in the real world, a process called ground truthing. Inaccurate predictions in public health, especially with false positives, can lead to mass hysteria about the spread of a disease.
AI for the common good
AI holds great promise for identifying where and how fast diseases are spreading. Increasingly, data scientists are using these techniques to predict the spread of diseases. Similarly, researchers are using these techniques to model how people move around within cities, potentially spreading pathogens as they go.
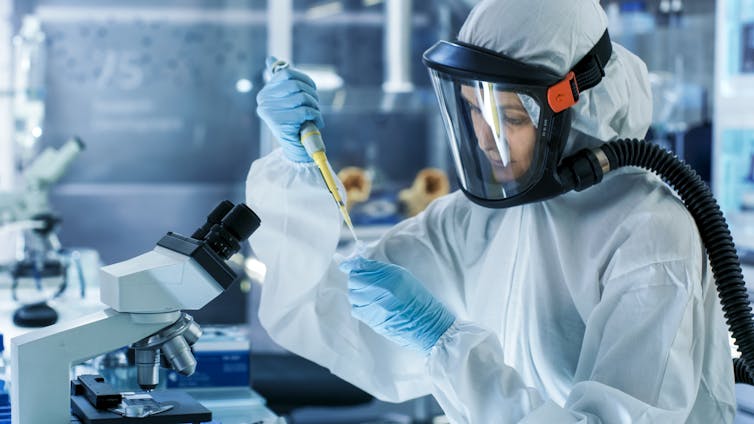
However, AI doesn’t eliminate the need for epidemiologists and
virologists who are fighting the spread on the front lines. For example,
BlueDot uses epidemiologists to confirm its algorithm’s results. AI is a
tool to provide more advanced and more accurate warnings that can
enable a rapid response to an outbreak. The key is bringing AI’s forecasting and prediction prowess to public health officials to improve their ability to respond to outbreaks.
Even if all else was perfect and AI were a technological silver bullet, the AI field would still face ethical challenges. We have to be more vigilant against phenomena like digital redlining, the computerized version of the practice of denying resources to marginalized populations, that can creep into AI outcomes. Entire regions or demographics could be sidelined, for example, from access to health care if the data used to train an AI system failed to include them.
In the case of AI models collating social media data, digital redlining can exclude entire populations with limited internet access. These populations might not be posting to social media or otherwise creating the digital fingerprints many AI models rely on. This could lead AI systems to make flawed recommendations about where resources are needed.
While researchers are continuously creating new AI algorithms, some of the foundational issues like understanding what’s going on inside the models, minimizing false positives and identifying and avoiding ethical issues are not well understood and require more research.
AI is a powerful tool for predicting and forecasting disease spread. However, it’s not likely to completely replace the tried-and-true combination of statistics and epidemiology first used when John Snow tracked down and removed the handle from the pump of a cholera-ridden water supply in 1854 London.
Vandana Janeja, Professor of Interim Chair of Information Systems, University of Maryland, Baltimore County
This article is republished from The Conversation under a Creative Commons license. Read the original article.- For additional UMBC Science and Technology stories, visit the UMBC News site.
- For additional stories about the UMBC community, visit the UMBC Magazine site.
- For additional COEIT stories, visit the COEIT site.
- For additional COEIT Research Highlights, including Publications Spotlights, visit the COEIT Research pages on the COEIT Dean's Office site.